What is The First Step to Spot Fake Reviews
To spot a fake review, looking for patterns in a product’s positive and negative reviews is important.
Fake positive reviews of products tend to be overly enthusiastic, lacking specific details about the product or service. They may also be written in a similar style or use the same phrases, indicating that they were generated by the same person or group.
On the other hand, fake negative reviews often contain exaggerated claims or focus on irrelevant aspects of the product or service. Pay attention to the rating distribution when shopping online and browsing the product reviews section.
Genuine reviews typically have a mix of positive and negative customer feedback, reflecting the diverse experiences of real customers. If a product has an overwhelming number of five-star reviews with few or no critical comments, it may be a sign that the reviews are not authentic.
Another flag for unreal reviews is a sudden influx of reviews within a short period, especially if the product or service has been available for a while. This could indicate that someone artificially buys phoney reviews to boost their ratings.
In this article, we will explore various ways to identify and avoid falling victim to fake online reviews and ratings, ensuring that you make well-informed decisions based on genuine experiences shared by real customers.
Do Two-thirds of All Products Really Have a Star Rating That Is Too Good?
There are more and more articles about fake reviews in the press and on various online news portals. This refers to various industries and their internet platforms, from gastronomy to travel to search engines. Mostly, it is about Booking.com, Tripadvisor, and Google. And, of course, about Amazon.
Interestingly, there is only some specialist literature or studies on the subject. Everyone knows that there are “fake” or purchased reviews; however, the extent and impact have been poorly documented.
The pioneers in uncovering and combating ” unreal reviews ” are usually consumer protection organizations or similar players. In the UK, this is “Which?”; for example, it is Stiftung Warentest in Germany.
As review experts, we at gominga are, of course, also intensively concerned with the topic of false reviews to provide our customers with advice and support.
Here, we would also like to refer to the chapter “Product Reviews on Amazon: Relevance and Recommendations for Companies” in the book “Amazon für Entscheider” (Springer Verlag, April 2020).
In the following sections, we have taken a recent article from Stiftung Warentest as an opportunity to examine the topic of “phoney reviews” and the procedure for identifying if a user review is fake. To do this, we used the analysis tool provider ReviewMeta analogously to evaluate Stiftung Warentest and applied an additional comparison evaluation with further review data.
In addition to our findings, we also provide further links on the topic, including current studies by “Which?” from the UK and Stiftung Warentest.
For several years, national and international studies have been conducted to prove the influence of reviews on consumers. We, too, have explained the value of customer reviews comprehensively.
A connection between reviews, price, and sales is often demonstrated. Therefore, it is understandable to a certain extent that there are attempts to influence the business positively by manipulating reviews.
Unreal reviews have become more and more common in attracting customers.
Fake reviews are most effective for lesser-known brands, products with few technical attributes, and low-priced items. Manipulators target star ratings, review quantity, rating distribution, and high-quality fake reviews. While platforms implement strict rules, manipulation persists through various means, from family and friends to a professional shadow industry using social media groups, payment services, bots, and review hijacking. Stiftung Warentest’s 2020 report detailed these practices, including buying reviews and analyzing them with ReviewMeta.
We used the report as an opportunity to examine the methodology, procedure closely, and results to compare them with another data set and replicate the analysis.
“The question and goal for us was: can you really easily identify reviews that have been bought? What is the percentage of reviews bought, and is there a difference between branded products and “no name”
Ways to Spot Fake Reviews
How can you recognize purchased reviews? What are the criteria? How can such a testing process be automated for large amounts of data?
One effective way to realise non-decent reviews is to identify patterns or similarities across multiple reviews for products.
If you notice that many reviews use similar language, phrasing, or specific keywords, it could be a sign that they are fake.
This is especially true if the reviews are posted within a short timeframe or if they are overly positive or negative without providing specific details.Also, pay attention to the name of the review writer. Unreal reviews tend to be written by profiles with generic names such as John Smith or Jane Doe.
Another red flag is a sudden influx of 5 stars or one-star reviews, particularly if they deviate significantly from the overall rating trend.
Genuine reviews tend to have a more balanced distribution of ratings. Numerous similar reviews could be fake as well.
Additionally, be cautious of reviews mentioning the review writer received a free product in exchange for feedback. While some companies do offer incentives for honest reviews, an unusually high number of such mentions could be a sign of fake reviews.
ReviewMeta is one of the most prominent tools on the market for identifying suspicious reviews on Amazon. The company first collects rating data from the various Amazon country platforms and then subjects it to an authenticity check.
For example, there are currently 5.3 million checked products on amazon.com and almost 650,000 products on amazon.de
of course, this means that it is a static check and not all current data can always be queried. In addition, the very large amount of data does not include all products and their reviews.
ReviewMeta mostly evaluates the top 100 products of different categories. These topseller lists then contain – sometimes more, sometimes less – a mixture of products from well-known brand manufacturers and low-priced no-name producers.
The tool is free, so anyone can query individual products and check for fake product feedback either at ReviewMeta directly or through a browser plugin. Finally, it should be mentioned that ReviewMeta doesn’t talk about “unreal reviews”; rather, it says, “ReviewMeta analyzes Amazon product feedback and filters out reviews that our algorithm detects may be unnatural.”
“ReviewMeta itself doesn’t talk about “fake reviews,” but rather, “ReviewMeta analyzes Amazon product reviews and filters out reviews that our algorithm deems unnatural.”
What Did gominga Investigate?
We used ReviewMeta’s tool to analyze and compare two different review datasets. The first test group comprises 45 products for which we know of purchased or “incentivized” reviews.
The second test group includes 110 products from brand manufacturers without purchased or “incentivized” reviews. All well-maintained assortments with consistently organically generated reviews and active customer support that responds to negative reviews and answers questions.
So our question was:
- Does ReviewMeta recognize purchased reviews?
- How many reviews does ReviewMeta mark as “unnatural”?
- How does the star value change after ReviewMeta sorts out “unnatural” reviews?
We were also interested to see if there was a difference between inexpensive, no-name products (often cell phone cases, accessories, cables, water bottles, etc.) and the products of brand-name manufacturers.
Calculating the star value is important for the analysis and the results. Both ReviewMeta and we can only calculate this using the arithmetic mean of the ratings. Amazon, on the other hand, uses an algorithm that considers various unknown factors. Therefore, there is usually a considerable discrepancy between the star value displayed on Amazon and the calculated value.
Amazon explains the calculation of the star value as follows:
“These models consider several factors, including, for example, how recent the review is or whether it is a verified purchase. They use multiple criteria to determine the authenticity of the feedback. The system continues to learn and improve over time. We do not consider customer reviews that do not have “Verified Purchase” status in the overall star-based rating of products unless the customer adds further details in the form of text, images, or videos”.
Both we and ReviewMeta only consider evaluations with text in our calculations. Although verified pure ratings without text are included in the overall average, these cannot currently be recorded externally, neither for us nor for ReviewMeta. These can only be determined by comparison if the product has already been recorded.
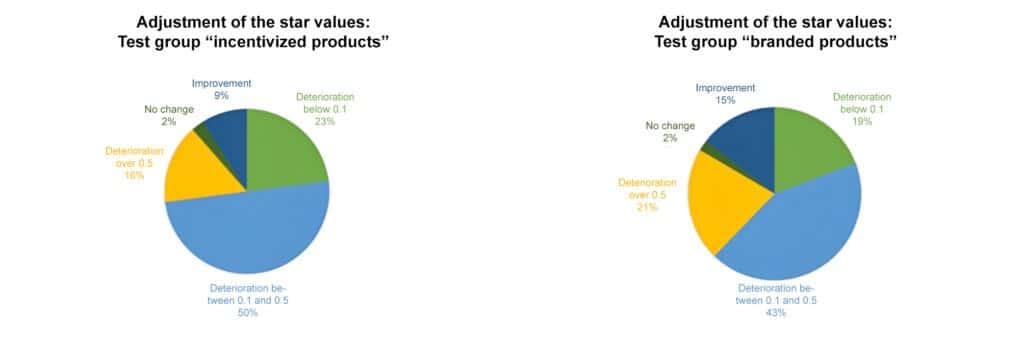
This adjustment is largely due to the different calculations by the star value shown on Amazon and the arithmetic mean of the recalculation.
For the products evaluated here, the fewest reviews (compared to the other clusters) were completely sorted out by ReviewMeta’s rules, i.e., the smallest adjustment occurred here.
At 16% for the test group with “incentivized” ratings and 21% for the products of the brand manufacturers, a larger change in the star value of over 0.5 can be determined. This would then result in a change in the graphical representation of the star value on Amazon. For example, 4 full stars are then displayed instead of 4 ½.
In 9% of the products with “incentivized” ratings and 15% of the products of brand manufacturers, the adjustments made by ReviewMeta even led to improvements in the rating. In other words, negative ratings are identified as “unnatural”.
There are no changes at all in 2% of the products.
Adjustment of the number of product reviews
The products in the test group with “incentivized” ratings had an average of 41 ratings before analysis by the ReviewMeta tool. The test group of branded products had 423.
There are many reasons for this. It could be argued that branded products are inherently better known among consumers and are, therefore, rated more often.
Furthermore, the product presentations of the brand manufacturers are presumably better maintained, and the topic of reviews and Q&A is actively managed.
On the other hand, the products in the test group with “incentivized” reviews have significantly fewer reviews, which is the original reason for buying reviews in the first place.
Sorting out and adjusting the quantitative number of reviews naturally leads to the possibility of finding many made-up reviews, which is consistently unequal for the two test groups.
In the test group with “incentivized” reviews, 61% had no changes at all in the number of reviews, i.e., 61% had no complaints at all or these did not lead to exclusion. In the case of branded products, this only affected 16%.
The Main Criteria of the Ratings Identified as “Unnatural”
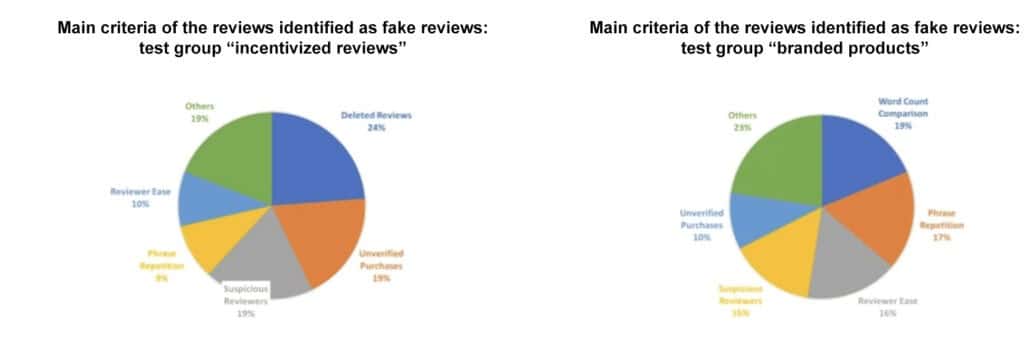
This adjustment is largely due to the different calculations by the star value shown on Amazon and the arithmetic mean of the recalculation.
For the products evaluated here, the fewest reviews (compared to the other clusters) were completely sorted out by ReviewMeta’s rules, i.e., the smallest adjustment occurred here.
At 16% for the test group with “incentivized” ratings and 21% for the products of the brand manufacturers, a larger change in the star value of over 0.5 can be determined.